Note to subscribers: I’m going to experiment with turning on paid subscriptions this week. The goal here is to see if Substack will promote this blog more if they can make money off of it. Please do not feel obligated to pay; the blog will go on regardless, and all posts will remain free.
As the old saying goes1, it’s easy to lie with statistics, but it’s much easier to lie without them. Which suggests that if you both include a statistic and leave one out, it’s even easier.
Ergo, there’s a quick and easy way to spot many statistical deceptions: if there’s only one stat, it’s a trap.
Example 1 - Leaving out the base rate.
17% of people who live near cell phone towers die of cancer.
I defy you to read that sentence without any part of you going “oh geez, there must be some connection!” It’s persuasive! But why?
That’s the sort of fact you would expect to see in an article about cell phone towers causing cancer. You wouldn’t expect to see it any other sort of article. So long as most of the stuff you’ve read was written in good faith, the pattern-recognizer in your brain will helpfully add in the missing steps. A statement can be literally true, but deceive someone via implicature: violating the assumption that what you’re saying is relevant. “Sorry I’m late; there was a crash on the highway” is deceptive if that crash had nothing to do with why you were late—your listeners fill in the missing connection, predictably converting your two truths into a lie.
The deception works if (and only if) your intuition about how many people die of cancer is either vague or misses low. If it seems plausible to you that the rate is only 4%, then 17% is a huge number—the risk is more than quadrupled.
People being fooled by a statistic don’t usually think that all through consciously. They just register that 17% looks like a big number in this context. And/or that it must be big, or why bring it up?
The statistical-industrial complex would have you believe that to refute this stat, you need a concept like “statistical significance” or “confounders” or something (even) more arcane. But in this case you don’t need to do any math. All you need to do is ask “And how many people in general die of cancer?”
In the wild:
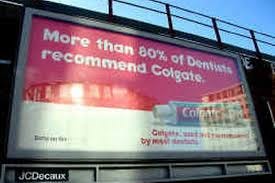
Example 2 - Mismatched units.
This program would cost over $10 million and only scan 2% of the area!2
This stat looks like it has two numbers in it, but it’s secretly only one. The implied claim is that the program in question has a low ratio of money-to-area-scanned. If we rewrote it to make that more explicit, “this program’s strategy spends $5 million per percent scanned,” it’d be more obvious what statistic is missing—what’s the ratio for the other options?
Or maybe the speaker is saying we shouldn’t bother trying to do the thing at all—that the alternative is nothing. In that case, the missing statistic we need is an estimate, in dollar values, of the benefits of scanning 2% of the area.
To spot this class of deception, you just need to check whether the two things being “compared” are of the same type. $10 million can’t be more than 2%, because dollars and square feet aren’t the same thing (nor are euros and square meters). In a valid use of statistics, you need to either be comparing dollars to dollars, or dollars-per-thingie to dollars-per-same-thingie.
(If you want to sound sophisticated, refer to this as “dimensional analysis” and don’t use the word “thingie.”)
In the wild:
Example 3 - Decoy comparisons.
85% of people who attend our seminar on how to become a flight attendant go on to become a flight attendant, compared to less than 1% of the general population!
This is an even sneakier way to leave out a statistic—include another one that seems like it should be relevant. The stat compares percentage-who-became-flight-attendants to percentage-who-became-flight-attendants, so it looks at first glance, and maybe even second glance, like it’s being honest.
Here, the missing statistic is “what percentage of people who try to become a flight attendant succeed”. That’s what to care about, because the goal is to figure out if, given that you’re trying to be a flight attendant, you should attend the seminar. You don’t care about the general population, only your own reference class. You could also try to compare like with like by “adjusting for confounders” or using “pairwise comparisons,” but that involves a lot of math and sketchy assumptions. If an argument doesn’t even do that, or does that when the relevant comparison stat seems like it should be easy to get, that’s a red flag.
In the wild:
Admittedly, statistics is a legit field.
There are plenty of ways to “lie with statistics” that this rule will miss. Ideally, you or someone you trust does actual math to the numbers presented before you actually believe they support the claim.
But I think you can catch most deceptions, intentional and unintentional, by spotting the missing number. If there’s a relevant statistic the writer probably could have included, but didn’t, it’s best to assume that it contradicts their claim.
Attributed to Frederic Mosteller but I couldn’t immediately confirm.
Or, similarly, “This program would cost only $200,000 and scan more than 99% of the area!”